What type of content do you primarily create?
If you're feeling overwhelmed by the flood of new AI tools promising to revolutionize your workflow, you're not alone.
The one that’s all over my social feeds lately: Notebook LM from Google, powered by its Gemini AI model. Marketed as a “personalized AI collaborator,” Notebook LM lets you ask specific questions about uploaded content, summarize information, and create useful outputs like FAQs, study guides, or briefings. So far, so nothing-I-can’t-do-in-ChatGPT.
But here’s the feature that’s causing all that commotion on my feeds. Notebook LM promises to turn any document into a 15- to 30-minute podcast in five minutes, at most. Sounds interesting—but is it for serious podcasters? Or just for someone who’s idea of a podcast is a memo read aloud by a robot? I decided to find out.
How does Notebook LM work?
In a conversation on the New York Times’ Hard Fork podcast, author Steven Johnson, Google's editorial director for the project, explained the vision behind Notebook LM. Johnson says they focused the product on two key use cases: producing podcasts from content nobody would usually podcast about, like “arcane City Council meetings,” and “personalized learning” to help students or professionals quickly master complex material.
The idea is that it’s easier to consume and remember that kind of dense or boring material by listening to a conversation. And turning it into a podcast means you can consume it while driving or walking the dog, which certainly sounds better than sitting hunched over a desk trying to slog your way through by reading.
Notebook LM is particularly intriguing for anyone swimming in documents, whether or not you’re interested in podcasting. The tool can handle a staggering 25 million pages (about 40 books’ worth) at once, so you can “converse” with not just one lengthy document, but a whole lot of them. Johnson also stresses that anything you upload stays private; it’s used only in your current session and doesn’t train the model.
To turn a document, or a few million of them, into a podcast, you use the audio overviews feature. Just upload your documents and then click "Notebook guide." There you'll see a section called "Audio Overview" where you can click Generate to create the podcast.
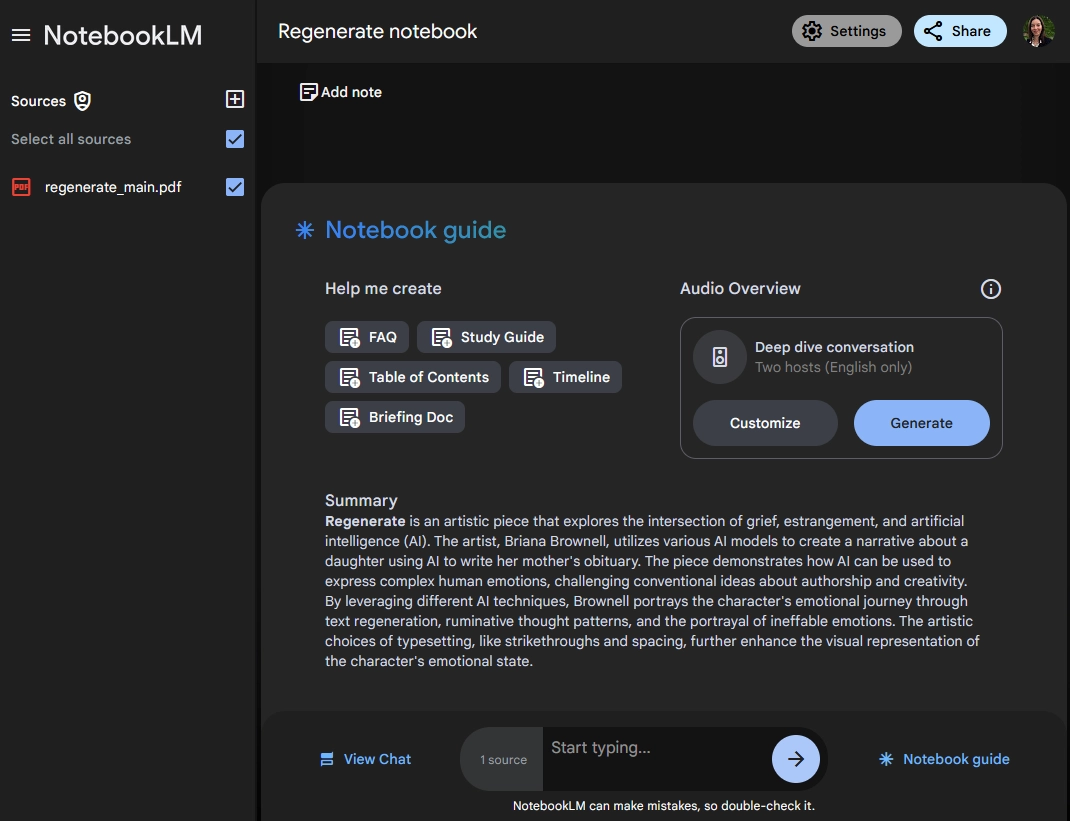
After about five minutes of AI magic, you'll have the overview, in the form of a podcast where a couple people talk through the contents of your document.
And that’s that. But before I talk about the results I got, it's worth spending a few minutes on what goes on behind the scenes, so you can really get what you want.
What Notebook LM does
On Hard Fork, Johnson explained how Notebook LM generates podcast scripts. You'll recognize it as a typical edit cycle using a method similar to the agent-based prompt framework we've written about before.
Here's what it does:
- Generate an outline, and then revises it (Johnson doesn't say exactly how it's revised)
- Generate a detailed script
- Critique the script (again, not clear how)
- Edit the script based on the critique
Once the script is ready, it’s narrated with AI voices. But they aren’t just reading word-for-word: Notebook LM incorporates natural "disfluencies" like pauses, filler words, and casual banter. Johnson says that’s “crucial because listening to two robots talk would be painful.” The AI voice tech also adds emphasis, hesitation, and intonation to make the audio more engaging and lifelike.
And there’s more on the horizon: Johnson hints that soon you’ll be able to provide more guidance, generate podcasts in multiple languages, and even interrupt the hosts with a question.
Hallucinations
The first audio overview I listened to was based on The Design of Everyday Things, by Rex Woodbury. Initially, I was impressed by the quality of the AI-hosted conversation—until about three minutes in. The hosts started discussing how France supposedly introduced a 10-franc coin similar in size to the 5-franc coin, which caused confusion. Sounded plausible. But then, the exchange took a strange twist:
Host 1: "In this case, people were primed to distinguish coins based on size, a cue that's deeply ingrained in our understanding of currency." Host 2: "Makes sense. I mean we don't even think about that with dollar bills, do we? A one dollar bill is way smaller than a hundred." Host 1: "Precisely."
Wait, what? I may be Canadian, but I know for certain that the U.S. hundred-dollar bill and one-dollar bill are exactly the same size.
Yep, that's a classic hallucination. Curious, I decided to fact-check the podcast against the actual book to see just how much NotebookLM had made up. Turns out, the shape of the story was accurate, but it was littered with inaccuracies. The actual confusion about francs wasn’t between the 10-franc and 5-franc coin, but between the 10-franc and half-franc coin, and that was why people were so mad.
While this error doesn’t completely derail the concept, it raises concerns about using these overviews as substitutes for reading the material. Creator, and listener, beware.
Can it teach you something?
Next, to test Johnson’s claim that Notebook LM can be a powerful learning tool, I put it to the test using one of the units from the Machine Learning course I teach at the University of Manitoba.
I uploaded the lesson text along with four supplementary readings for the week. I asked Notebook LM to focus on the primary material, as the additional content was lengthy and I wanted it to stick to the core concepts. It created a 17-minute podcast, beginning with:
Host 1: “Today we’re taking a deep dive into Unit 5 of this machine learning course, focusing on Prediction and Classification...”
Host 2: “Sounds exciting!”
I appreciated Host 2’s enthusiasm, but as the podcast continued, I found myself disappointed. It strayed off course, introducing several hallucinations—including a detailed discussion on medical diagnosis and image analysis, which were nowhere in my materials. This is a well-known issue with AI-generated summaries, but it’s particularly concerning from a tool that’s supposedly built for learning. If you’re planning to use Notebook LM in an educational setting, approach it with caution, and not as a substitute for the original content.
That said, it did manage to explain certain concepts clearly and concisely—and it's always a plus to have the same concepts explained multiple ways. So I still think it’s useful.
Arcane city council meetings
Johnson used the “arcane city council meeting” as an example of another NotebookLM use case: creating podcasts from material no one would ever typically make a podcast about.
So I used it to make a podcast about the most universally unappealing document I could think of: The Bank of Montreal's Annual General Meeting of Shareholders in 1873. For good measure, I also fed it the same meeting 50 years later in 1923.
The result? Absolutely glorious.
The AI hosts pulled snippets from each meeting, contrasting and comparing the details in a way that was both insightful and surprisingly entertaining. For a “use case” designed for the most boring of documents, I’d rate it extremely useful. With a quick listen, I could decide what parts were interesting enough to dive deeper into.
Next, I tested Notebook LM on a few other types of documents, including a UN report, an academic paper, and even my own articles. I found that the more organized the content, the better the AI’s output. For example, the UN A4P+ report was neatly structured into seven priorities, and the AI followed this structure closely, summarizing each section in a way that captured the essentials well. However, if the document was dense or complex, the AI would cherry-pick points, so I didn’t always get a complete summary.
The audio overviews of my articles were solid, though not quite as nuanced as if I’d narrated them myself. But for a five-minute generation time, they were impressive.
Academic papers, on the other hand, were hit-or-miss. When the paper was well-structured, Notebook LM did a decent job of distilling the main points. But with less organized articles, it struggled, highlighting the irony of needing to organize content before generating an “automatic” overview. After all, if you’ve already done the work of structuring it, do you really need the overview?
Is Notebook LM useful for podcasters?
As someone always looking to save time, I was curious to see if Notebook LM could help speed up script creation for a podcast episode. To test it, I uploaded the raw materials for an episode of a limited series—a newspaper article and my notes.
To my surprise, Notebook LM did a solid job of identifying the main themes, producing a decent introduction, and even generating well-phrased questions and discussion points. That said, it has its own distinct “voice,” and if this style doesn’t match your podcast’s tone, its utility may be limited. Still, there were some good takeaways I’ll keep in mind when I finally get to that episode.
One interesting quirk: if you generate a podcast on the same material multiple times, the output will differ each time. This could be either a feature or a limitation, depending on how much consistency you need.
In terms of document querying, Notebook LM works about as well as other tools, but that extra-large context window is a big bonus—it lets you dig through way more content to find what you need.
Can Notebook LM handle 40 Books?
Notebook LM claims it can handle up to 40 books. Realistically, though? Not quite.
Maybe it’s a bit unfair to expect the system to compress multiple books into a 15- to 30-minute podcast. At best you'll get a few interesting points. However, I found this problem was, at times, surprisingly bad. When using a lot of source material, the examples mentioned were incomplete or didn't quite tease out the point the author was trying to make.
Let me show you what I mean—this example is long, so feel free to skip it if you just want to get to the goods—but it does give you a sense of the kinds of errors it is making so it might help you be more vigilant about hallucinations.
Example: I created an overview podcast based on The Design of Everyday Things. The AI summary mentioned how nurses are often interrupted on the job, which can make it frustrating to work with electronic medical records that log them out automatically.
Here’s the AI’s version of that story:
"And that brings us to another crucial consideration. Designing for human limitations. We all have them...Good design takes these limitations into account and creates systems that work with our natural tendencies rather than against them. For example, consider electronic medical records, systems that constantly log out nurses due to brief interruptions. Those interruptions are a normal part of their job, so the system should be designed to accommodate them."
The AI hosts moved on, noting that sometimes adding security can ironically reduce security—but without actually making that point.
Here’s what the book actually says:
"I have seen nurses write down critical medical information about their patients on their hands because the critical information would disappear if the nurse was distracted for a moment by someone asking a question. The electronic medical records systems automatically log out users when the system does not appear to be in use. Why the automatic logouts? To protect patient privacy. The cause may be well motivated, but the action poses severe challenges to nurses who are continually being interrupted in their work by physicians, co-workers, or patient requests. While they are attending to the interruption, the system logs them out, so they have to start over again. No wonder these nurses wrote down the knowledge, although this then negated much of the value of the computer system in minimizing handwriting errors. But what else were they to do? How else to get at the critical information? They couldn’t remember it all: that’s why they had computers."
The AI’s summary touched on the concept but missed the heart of it: nurses were forced to work around a privacy system in a way that actually created greater privacy risks. If I were writing a script on that passage, there's no way I would have excluded that crucial point.
Notebook LM, like most tools that compress books into short summaries, is helpful in one specific way: it’s a good way to decide whether you actually want to read the book. When a book is packed with rich content, there’s just too much to fully capture in a 30-minute audio overview. But if you’re just trying to get a general sense of the ideas, it can give you enough to know if it’s worth diving into.
Random in, absurdity out
The audio overview feature works best on well-organized, clear content. But since you can put literally anything into the notebook, the results can get...strange.
For instance, when Kevin Roose feeds it a credit card statement, the AI hosts start shaming him for his reliance on Uber, suggesting he should think about taking the bus.
But it gets weirder: someone fed it the infamous “chicken paper,” a gag academic paper by Doug Zongker where every word is chicken. The AI hosts went off on an absurd and hilarious philosophical tangent, musing on the idea that “chicken could be its own language,” and advising listeners to “forget dictionaries, forget grammar—we can explain everything with chicken.” It’s quite funny.
Others have played similar gags on NotebookLM. Useful? Not always. Entertaining? Every time.
Does NotebookLM live up to the hype?
I started this research as a skeptic, doubtful that NotebookLM would be very useful for podcasters or creators, but I came away pleasantly surprised. The ability to create a podcast-style overview from any document proved more helpful than I expected. While the 25-million-page context window might be overhyped, Notebook LM still excels at quickly generating useful overviews of shorter documents. Even with its hallucination issues, it’s earned a place in my roster of favorite AI tools.
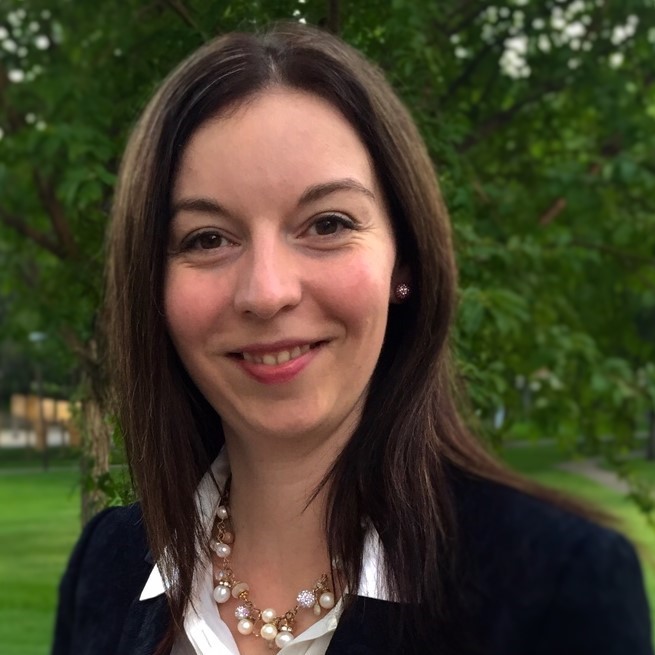