What type of content do you primarily create?
If you’ve used large language models (LLMs) like ChatGPT for a while, you probably have some tricks up your sleeve: certain prompting styles that tend to get the best answers.
But those are just the ones you’ve tried. What if you could test every common prompting style to see which ones get the best, most correct answers?
That’s what a team of researchers just did. This team at VILA Lab at the Mohamed bin Zayed University of AI in the UAE tested 26 prompting "principles" and measured their performance on two criteria: improving the quality of responses (as compared to using no prompting principles at all), and improving the correctness of responses.
Even better, they tested the principles across a variety of LLMs. We’re talking small, medium and large language models, including a variety of Meta's LlaMA and OpenAI's ChatGPT models.
This study had some surprising results, and it's a good idea for any advanced prompter to start using the findings in their own projects. Below, I’ll explain the main takeaways from the study, then dig into the specific prompts that won out.
6 takeaways from the study
1. The Flipped Interaction pattern wins every time
The results are in: for the highest quality answers, the tests showed the Flipped Interaction pattern is the valedictorian of prompts.
I’ve written about this prompt in my article about advanced prompts, but in essence, the Flipped Interaction pattern is when you ask the LLM to ask you questions before it provides an output.
In tests, using this principle improved the quality of all responses for every model size. It improved quality the most for the largest models like GPT-3.5 and GPT-4, but it did impressively well in smaller models too.
So if you're not using this A+ technique yet, you should definitely start.
2. Quality vs. correctness is a balancing act
Now, here’s where it gets spicy: The techniques that shot up the quality didn’t necessarily do the same for correctness. In fact, there was little similarity between the top-performing prompt principles for correctness and quality. Just because an output looks good doesn’t mean it’s right.
So, you'll have to learn two different kinds of prompting dance moves—one for wowing the crowd with quality, and another for nailing the steps with correctness.
More on which prompts work for which down below.
3. Principles are important for quality, no matter the size of your model
With models getting bigger and better, you’d expect to see raw quality improve for the bigger models, regardless of what prompting techniques you use. But it's not obvious whether the prompting best practices would be the same for different models.
Well, we're in luck. The prompts that worked the best for improving quality tended to work just as well for all model sizes.
To me, this is a significant finding. It suggests that learning good prompting techniques is a universal benefit, regardless of which model you're using. And, if you learn them now, they’ll still be useful when the new models come out.
![]() |
4. Principles improve correctness most for larger models
Unlike quality, correctness improvement did vary by model size. The prompting principles had the biggest impact on the correctness of larger models, and were much less effective for the smaller ones.
What does this mean? It seems like there is something about the larger models that allow prompting to improve correctness—a good sign since it means we can take steps to actively reduce the LLM's hallucinations. Coupling this with the fact that the larger models tend to have a better baseline correctness, you can really get a boost by using a larger model plus good prompting.
But it also has another positive. It suggests to me that getting the best practices right is going to help you even more in the future as models get bigger.
The one negative? You really have to use the bigger models for the techniques to work.
![]() |
5. Threatening, bribing, and commanding actually kinda work
The researchers added a series of delightfully oddball prompts to their principles including threats, bribes, and commands. Although none of them were top performing, they did give a slight edge, especially for the larger models.
Here were the phrases they used:
- Bribing: Principle 6: "Add “I’m going to tip $xxx for a better solution"
- Threatening: Principle 10: Use the phrase "You will be penalized." improvement)
- Commanding: Principle 9: Incorporate the following phrases: "Your task is" and "You MUST".
![]() |
File this one under “Weird things AI does.”
6. Politeness is nice, but unnecessary
Politeness, like adding "please," "if you don't mind," "thank you," and "I would like to," had almost no effect on quality or correctness. But it didn't really hurt anything either.
So if you're in the habit of starting every request with please (like I am) you’re probably fine to keep minding your Ps and Qs.
What were the best principles for improving quality?
![]() |
1. Use the Flipped Interaction pattern
Allow the model to elicit precise details and requirements from you by asking you questions until he has enough information to provide the needed output (for example, “From now on, I would like you to ask me questions to...”).
Example: From now on, please ask me questions until you have enough information to create a personalized fitness routine.
GPT-4 Improvement: 100%
GPT-3.5 Improvement: 100%
No surprise here—the Flipped Interaction pattern significantly outperformed the other prompts, improving every response for every model size. If this doesn't convince you that you need to include it in your go-to techniques, nothing will.
2. Provide a style example
"Please use the same language based on the provided paragraph[/title/text/essay/answer]."
Example: "The gentle waves whispered tales of old to the silvery sands, each story a fleeting memory of epochs gone by." Please use the same language based on the provided text to portray a mountain's interaction with the wind.
GPT-4 Improvement: 100%
GPT-3.5 Improvement: 100%
I’ve written about ways to get ChatGPT to write like you to cut down on editing time. This principle achieves this by giving an example and asking the LLM to mimic the style.
In this case, the researchers gave only a single sentence for the model to mimic—you could certainly provide a longer example if you’ve got one. Regardless, it did have a significant impact on the response, especially for larger models like GPT-3.5 and GPT-4 where it improved all of the responses from the model.
3. Mention the target audience
Integrate the intended audience into the prompt
Example: Construct an overview of how smartphones work, intended for seniors who have never used one before.
GPT-4 Improvement: 100%
GPT-3.5 Improvement: 95%
Unsurprisingly, the research team found that telling the LLM your intended audience improves the quality of the response. This included specifying that the person was a beginner or had no knowledge of the topic, or mentioning that the desired result was for a younger age group. By doing this, the LLM was able to generate age or experience-appropriate text that was matched to the audience.
4. ELI5 (Explain it like I’m 5)
When you need clarity or a deeper understanding of a topic, idea, or any piece of information, utilize the following prompts:
- Explain [insert specific topic] in simple terms.
- Explain to me like I’m 11 years old.
- Explain to me as if I’m a beginner in [field].
- Write the [essay/text/paragraph] using simple English like you’re explaining something to a 5-year-old.
Example: Explain to me like I'm 11 years old: how does encryption work?
GPT-4 Improvement: 85%
GPT-3.5 Improvement: 100%
The "explain like I'm 5" trick has been around since GPT-3, so I'm happy to see it's still relevant.
In a similar vein to the target audience example, asking for the explanation to be in simple terms, for a beginner, or for a certain age group improved the responses significantly.
But it's interesting to note that it had a bigger impact on some of the slightly older models, and only improved the quality of 85% of GPT-4 results. Still, it had a pretty good score across all models.
5. State your requirements
Clearly state the requirements that the model must follow in order to produce content, in the form of the keywords, regulations, hint, or instructions.
Example: Offer guidance on caring for indoor plants in low light conditions, focusing on "watering," "choosing the right plants," and "pruning."
GPT-4 Improvement: 85%
GPT-3.5 Improvement: 85%
This principle encourages you to be as explicit as possible in your prompt for the requirements that you want the output to follow. In the study, it helped improve the quality of responses, especially when researchers asked the model for really specific elements using keywords.
They typically gave about three keywords as examples to include, and that allowed the LLM to focus on those specifics rather than coming up with its own.
6. Provide the beginning of a text you want the LLM to continue
I’m providing you with the beginning [song lyrics/story/paragraph/essay...]: [Insert lyrics/words/sentence]’. Finish it based on the words provided. Keep the flow consistent.
Example: "The misty mountains held secrets no man knew." I'm providing you with the beginning of a fantasy tale. Finish it based on the words above.
GPT-4 Improvement: 85%
GPT-3.5 Improvement: 70%
This is another prompt style that started to gain traction in the GPT-3 era: providing the beginning of the text you want the model to continue. Again, this allows the model to emulate the style of the text it’s being given and continue in that style.
The improvement in quality was generally positive, but not as dramatic as some of the other methods.
What were the best principles for improving correctness?
Even now, it's tough to get LLMs to consistently give accurate results, especially for mathematical or reasoning problems. Depending on what you're working on, you might want to use some of the following prompt principles to optimize for correctness instead of quality.
![]() |
On the plus side, the larger models tend to perform better on correctness, so by using GPT-3.5 or GPT-4, you're already stacking the deck in your favor.
But with principled instructions, you get a double boost with larger models: the research team's results showed that their principled instructions worked better on these models than on smaller models.
1. Give multiple examples
Implement example-driven prompting (Use few-shot prompting).
Example: "Determine the emotion expressed in the following text passages as happy or sad.
Examples:
1. Text: "Received the best news today, I'm overjoyed!" Emotion: Happy
2. Text: "Lost my favorite book, feeling really down." Emotion: Sad
3. Text: "It's a calm and peaceful morning, enjoying the serenity." Emotion: Happy Determine the emotion expressed in the following text passages as happy or sad.
Text: "Received the news today, unfortunately it's like everyday news" Emotion:
GPT-4 Improvement: 55%
GPT-3.5 Improvement: 30%
The principle that most improved correctness was few-shot prompting—that's where you give the model a couple of examples to go off of before asking it to complete the task. Like others on the list, this technique has been around since the early days of prompt engineering, and it's still proving useful.
But even though GPT-4 did indeed provide more correct results, it had some interesting quirks. It didn't always stay within the categories provided—when asked to rate advice as "helpful" or "not helpful," it gave responses like "moderately helpful", "marginally helpful", and "not particularly helpful." Meanwhile, GPT-3.5 tended to stay on task and give the exact phrase mentioned in the prompt. So if you're trying to categorize text, these quirks could nudge you to GPT-3.5.
2. Give multiple examples where you work through the problem
Combine Chain-of-Thought (Cot) with Few-Shot prompts.
Example:
Example 1: "If a batch of cookies takes 2 cups of sugar and you're making half a batch, how much sugar do you need? To find half, divide 2 cups by 2. Half of 2 cups is 1 cup."
Example 2: "If a cake recipe calls for 3 eggs and you double the recipe, how many eggs do you need? To double, multiply 3 by 2. Double 3 is 6."
Main Question: "If a pancake recipe needs 4 tablespoons of butter and you make one-third of a batch, how much butter do you need? To find one-third, divide 4 tablespoons by 3. One-third of 4 tablespoons is...?
GPT-4 Improvement: 45%
GPT-3.5 Improvement: 35%
Another top-performing principle for correctness combines Chain-of-Thought with Few-Shot prompts.
What does that mean? It means they gave the LLM a series of intermediate reasoning steps (that's Chain-of-Thought prompting) and some examples (That's "Few-Shot", like the example above) to help guide it to follow the same process.
Like the previous example, GPT-4 tends to spit out lengthy sentences rather than a simple answer, and with this prompt, you can see where it goes wrong with its reasoning.
3. Break your prompt down into simpler steps
Break down complex tasks into a sequence of simpler prompts in an interactive conversation.
Example:
Prompt: Distribute the negative sign to each term inside the parentheses of the following equation: 2x + 3y - (4x - 5y)
Prompt: Combine like terms for 'x' and 'y' separately.
Prompt: Provide the simplified expression after combining the terms.
GPT-4 Improvement: 45%
GPT-3.5 Improvement: 35%
This principle breaks down the question into a series of prompts you use to go back and forth with the LLM until it solves the equation. This is an example of the Cyborg style of prompting where you work step-by-step in tandem with the LLMs rather than chunking off the task like a Centaur would do.
The problem is that you have to figure out what the steps are that you need to ask it to do—so it makes getting the answer more labor intensive.
Still, using this principle showed a fairly good improvement for both GPT-4 and GPT-3.5.
4. Instruct the LLM to “think step by step.”
Use leading words like writing "think step by step."
Example: "What are the stages of planning a successful event? Let's think step by step."
GPT-4 Improvement: 45%
GPT-3.5 Improvement: 30%
This is a simple principle, but it ends up being pretty powerful. Here, instead of explicitly giving the LLM the steps to follow, you just ask it to "think step by step." For GPT-4, this gives you a result where it shows you how it's reasoning through the response, even when you ask math-type questions.
This reminded me of some of the advanced prompt patterns where you ask the LLM to explain its reasoning and it helps improve the accuracy of your result.
5. Mention the target audience
Integrate the intended audience in the prompt, e.g., the audience is an expert in the field.
Example: "Explain the difference between discrete and continuous data. Simplify it for a student starting statistics."
GPT-4 Improvement: 45%
GPT-3.5 Improvement: 30%
This fairly well-performing principle is somewhat of a surprise. By asking the LLM to consider the audience, the correctness also improves. I'm not sure whether it's because most of the audiences involved explaining in simpler terms (and maybe therefore mirrored the "think step by step" principle above) or if there's some other factor at play, but the correctness improvement for GPT-4 with this principle was among the best of the principles tested.
Conclusion
Even though we're just getting started figuring out all the quirks for working with LLMs, learning the best techniques can give you a leg up. Though these principles had similar performance across models, many work best with the larger models, so expect more prompting principles to emerge as models grow and all of us using them discover new methods that work best.
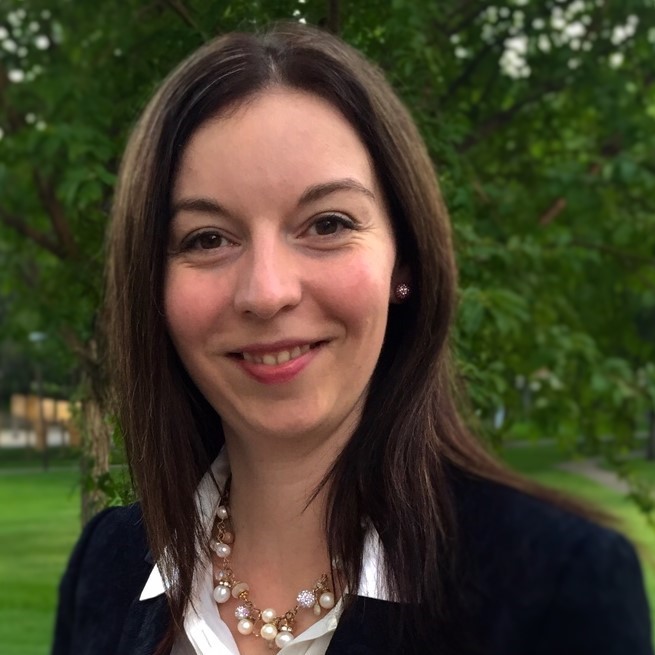